A Case for AI in Strategic Planning
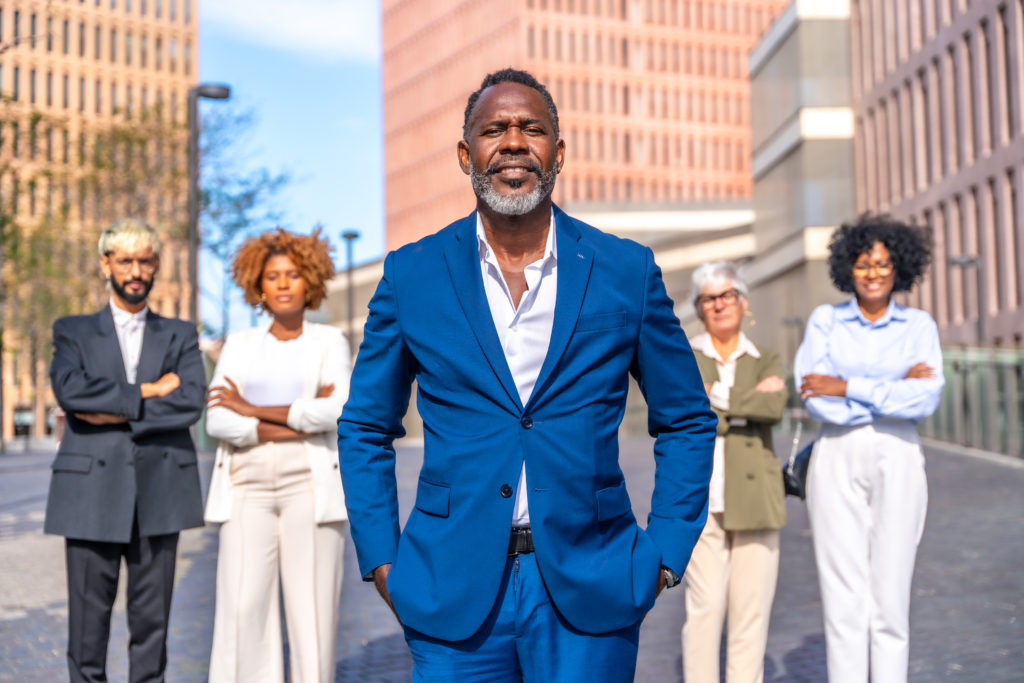
In writing my third book, only one published as yet, about cultural diversity and its profound impact on organizational performance, I aimed to substantiate my claims with data, visualizations, and reports. The objective is to demonstrate how well-implemented diversity programs can result in potential 35% increases in revenue, efficiency, and effectiveness. Leveraging data science and machine learning, I was able to generate a preliminary comprehensive dataset of 5,000 employees, run strategic scenarios, and identify a few key factors driving success. I need 32k executives, managers, and employees because why work with a sample when you can generate the full set.
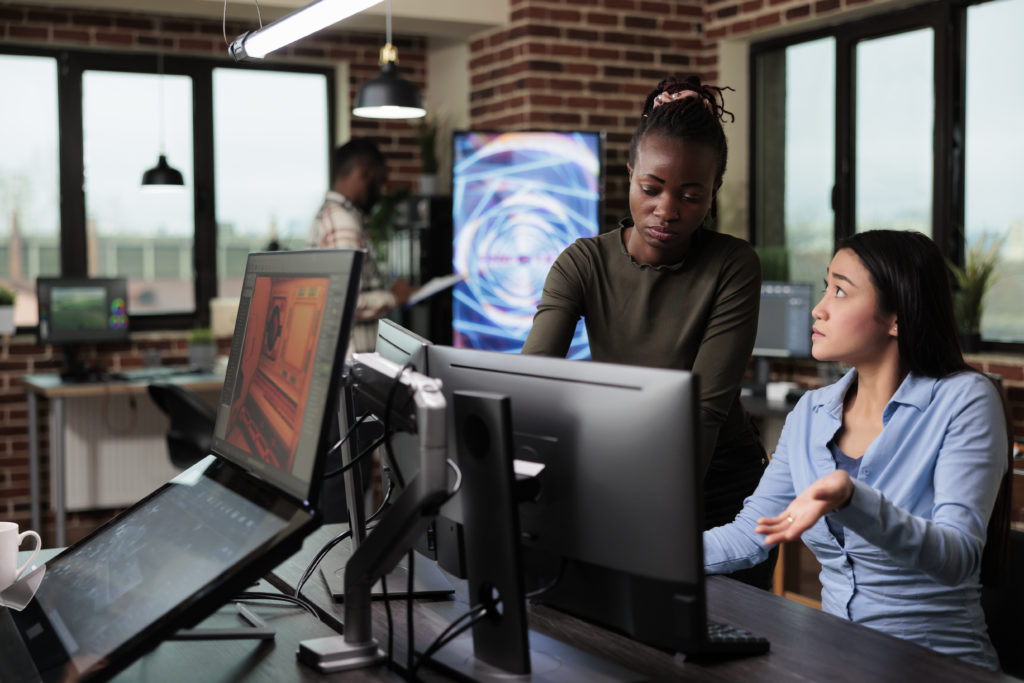
Harnessing Data Science and Machine Learning
- Generating Comprehensive Datasets:
- Using data science tools like Python and Jupyter Notebooks, I intend to create a dataset encompassing a full company roster of 32,000 rows. This dataset includes employees, managers, and executives broken down into random demographics.
- The richness of this dataset would allow for a detailed analysis of the workforce composition, identifying patterns and trends that could inform diversity initiatives in the context of the strategic vision.
- Running Strategic Scenarios:
- With machine learning models, I will simulate various scenarios based on strategic objectives and initiatives to occur over a 3-5 year period; I’ll run these over the course of days and months. These scenarios will be designed to evaluate the impact of proposed diversity initiatives on key performance metrics such as revenue, efficiency, and effectiveness. Some feature (variable) engineering may be required for optimum performance.
- By plotting the features (variables) most responsible for the outcomes, I could pinpoint the critical success factors that would help drive success. I say help because leadership is the one true driver of success, meaning, everything could be handed to you but without leadership– outcomes are less certain!
- Feature Engineering for Predictive Insights:
- Feature engineering enables one to transform raw data into meaningful features that improve the predictive power of any model.
- This process helps in identifying the variables that are most likely to influence the target (label) outcomes, providing actionable insights for decision-making.
The Importance of Real Data in AI Models
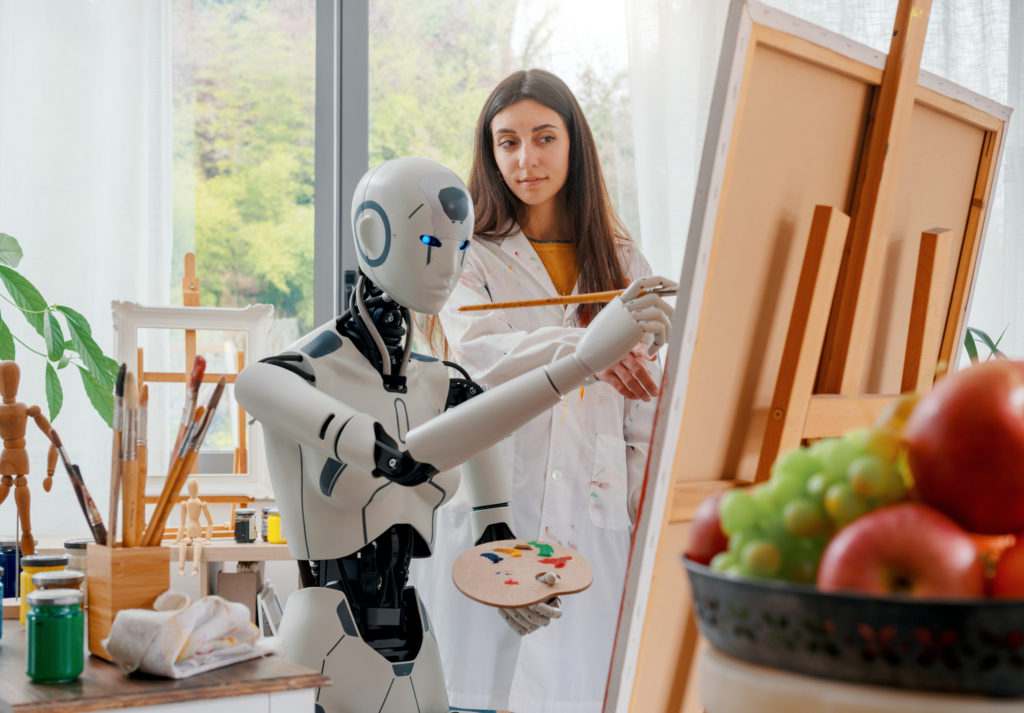
A significant issue in the field of AI is the debate over the use of real data for training models. Some argue that using real data is akin to plagiarism. However, this perspective overlooks the essence of education and learning, which is fundamentally about building upon existing knowledge. I think the issue is more about job security than anything else!
- Real Data Ensures Accuracy:
- AI models trained on real data are more accurate and reliable because they reflect real-world scenarios. Without real data, models tend to lack the necessary context to make sound predictions. I said tend, because AI has algorithms that can detect errors in prediction and self correct or adjust. It’s just that the process could be managed better with cooperation, but honestly, none needed.
- In the context of cultural diversity initiatives, using authentic data helps ensure that the models can more accurately simulate the impact of proposed programs and provide valid recommendations; it’s actually been done instead of speculated.
- Learning and Building on Existing Knowledge:
- The argument against using real data as plagiarism undermines the very foundation of education in general. Supposedely, learning involves absorbing information, understanding it, applying, evaluating, and creating it to new situations. This is a core element of Bloom’s Taxonomy!
- When an AI answers a question, it synthesizes information from vast datasets, including a long thread and conversation I might have been having with the AI for several weeks. I don’t need answers to outside questions– I need answers to my questions. And, since they have not been privy to public disclosure, how can they be plaigerized? If the specific question has never been asked before, the answer is not plagiarized but rather a novel synthesis based on existing knowledge and the current thread I’m engaged in with the AI–- a private conversation.
- Ethical Considerations:
- Ensuring that data is used ethically is crucial. This involves obtaining data legally, respecting privacy, and mitigating biases. I would argue for job preservation purposes, it’s in the best interest of some to slow that process down and make it illegal to use real data. One might also argue that when you use AI data, it should also be illegal and maybe even charged for its use. I don’t advocate this but what’s good for the goose is good for the gander.
- Properly sourced and used real data enhances the credibility of AI models and the decisions they inform. Besides, why can’t we all just get along?
Conclusion
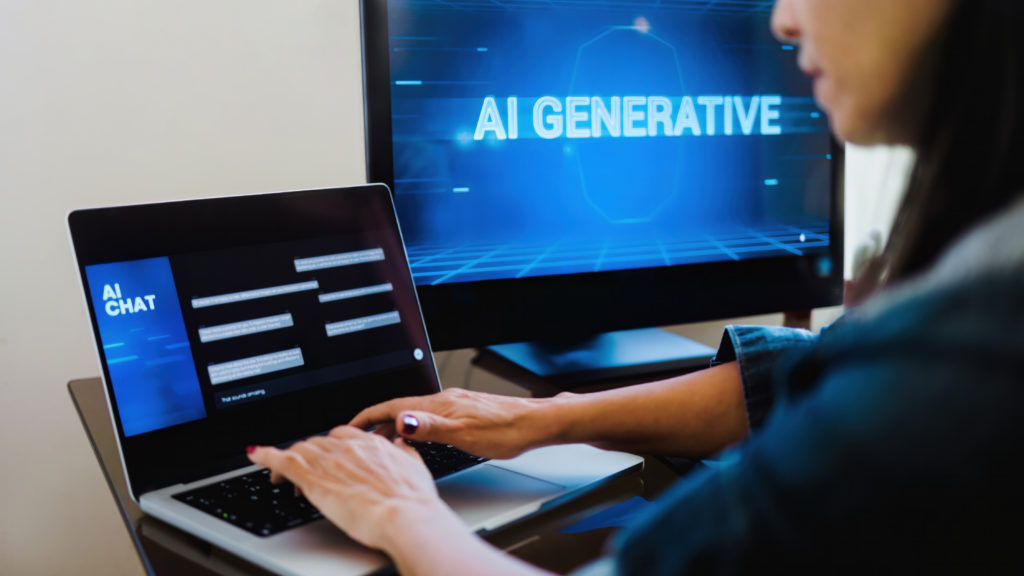
Data science and machine learning offer powerful tools for developing and evaluating cultural diversity initiatives. By generating comprehensive datasets, running strategic scenarios, and performing feature engineering, organizations can gain predictive insights that inform their diversity strategies and achieve the strategic vision. The use of real data is essential for the accuracy and reliability of these models. Dismissing real data as plagiarism overlooks the principles of learning and the critical role of data in driving informed decisions.
In the realm of AI and machine learning, it is essential to embrace real data while ensuring ethical practices. This approach not only enhances the performance of AI models but also supports the development of initiatives that can lead to significant improvements in revenue, efficiency, and effectiveness.