A Visionary Framework for Long-Term AI Systems
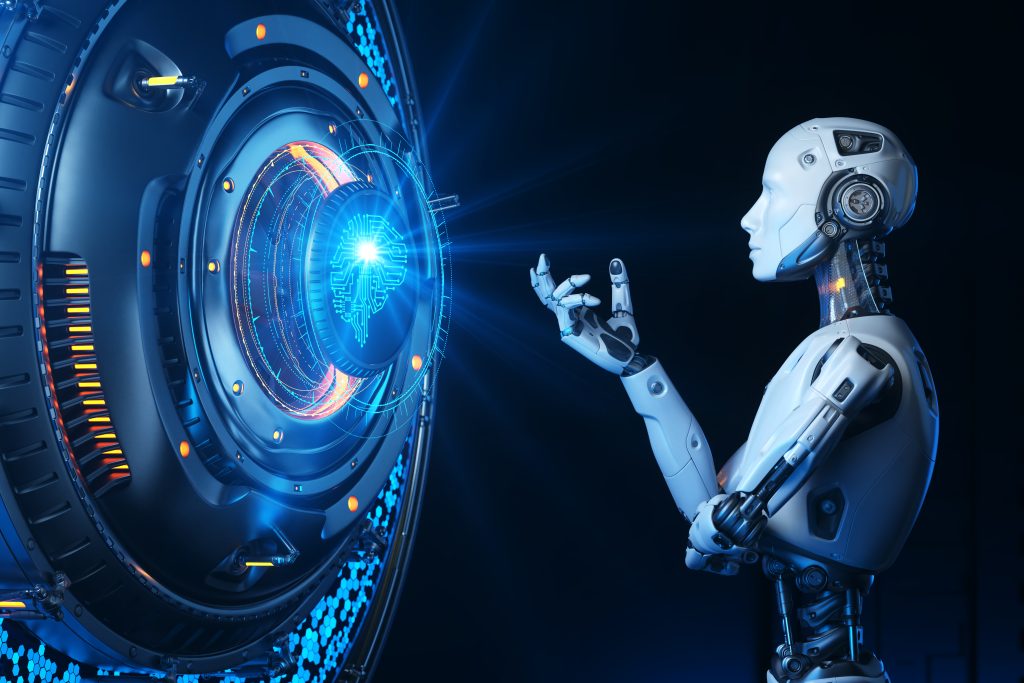
Introduction
Artificial Intelligence (AI) systems have transformed the way we interact, learn, and work, yet much of their design has been rooted in short-term optimization. Current AI systems, especially text-based ones like ChatGPT or Google Bard, are designed for ephemeral sessions, meaning they lack continuity in their “understanding” of user needs or contexts over time. This limitation poses a significant challenge in achieving meaningful long-term goals, especially when addressing complex issues like intercultural competence (IC).
What if AI systems could engage in long-term learning, building memory and applying insights across multiple interactions? This blog introduces an innovative framework to make this vision a reality, leveraging modernized Kalman filters to create adaptive, long-term AI models. The proposed framework integrates Inventory of Cross-Cultural Sensitivity version 2 (ICCSv2) metrics, facilitating AI systems capable of aligning with intercultural competence benchmarks while revolutionizing user-AI interactions.
The Problem: The Limitations of Short-Term AI Models
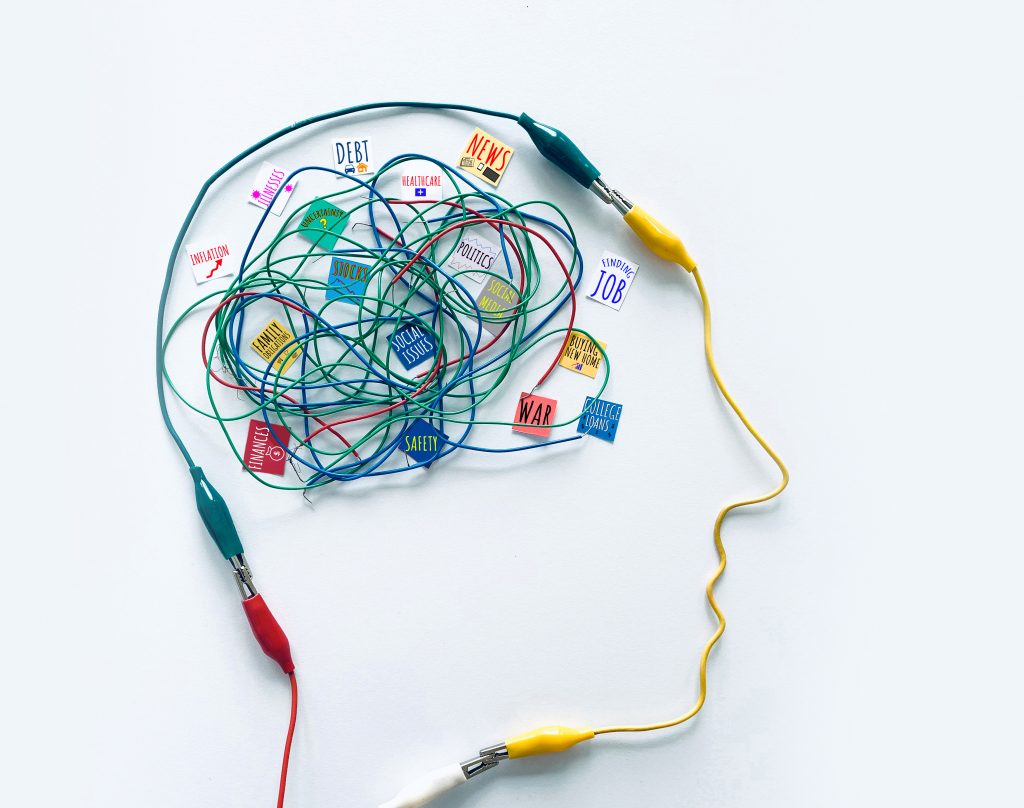
Wiring the long-term memory in the brain. Credit Envato.
Modern AI systems operate with what can be described as “sessional amnesia.” Each user interaction is treated as an isolated event, with minimal or no retention of prior inputs or insights. While this design safeguards privacy and ensures operational efficiency, it falls short in scenarios where cumulative learning is critical.
For example:
- Cultural Sensitivity: AI systems cannot adapt or improve their responses based on user interactions over time.
- Personalization: Short-term models cannot recognize nuanced user preferences or needs, leading to generic outputs.
- Ethical and Inclusive AI: Without the ability to evolve, AI systems cannot refine their responses to better align with intercultural competence goals.
This short-term design perpetuates limitations in deploying AI effectively in education, business, or even global citizenship initiatives where cultural understanding and memory matter deeply.
The Innovation: Long-Term AI Systems with Modernized Kalman Filters
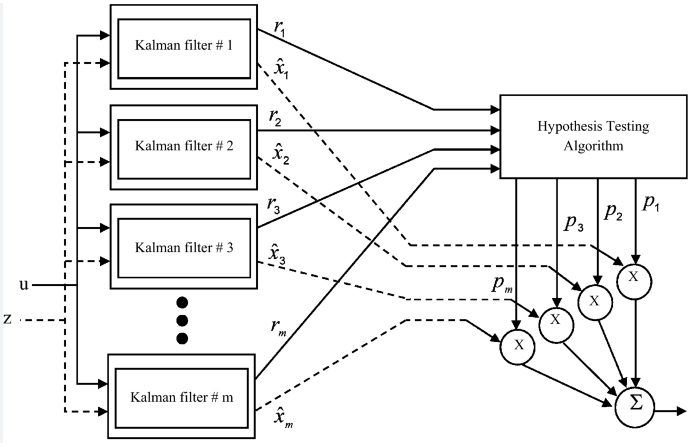
Kalman filters can be calibrated to parameters with go-no-go data and then be autonomous. https://images.app.goo.gl/7C6ZUZ9Dm3Z9e47e6
The proposed solution introduces a groundbreaking use of modernized Kalman filters—a mathematical algorithm traditionally used in navigation systems for real-time error correction and prediction. By adapting Kalman filters for AI systems, this framework allows for dynamic adjustments, continuous learning, and iterative calibration over extended periods.
How It Works:
- Adaptive Memory Storage: Kalman filters enable AI systems to store, update, and prioritize data from previous sessions. For example, an AI system interacting with a student learning intercultural competence can retain progress and tailor future responses.
- Iterative Calibration: The filter dynamically recalibrates the AI system based on user inputs, ICCSv2 metrics, and contextual variables, ensuring responses remain culturally sensitive and aligned with long-term goals.
- Personalization Beyond Sessions: By leveraging long-term data, AI systems can adapt to individual user preferences while maintaining ethical safeguards like transparency and accountability.
- Scalable Intercultural Competence Metrics: The integration of ICCSv2 subscales—Cultural Inclusion, Cultural Anxiety, Cognitive Flexibility, and Behavioral Integration—provides a robust framework for training AI systems to navigate intercultural dynamics.
Implications for AI Systems
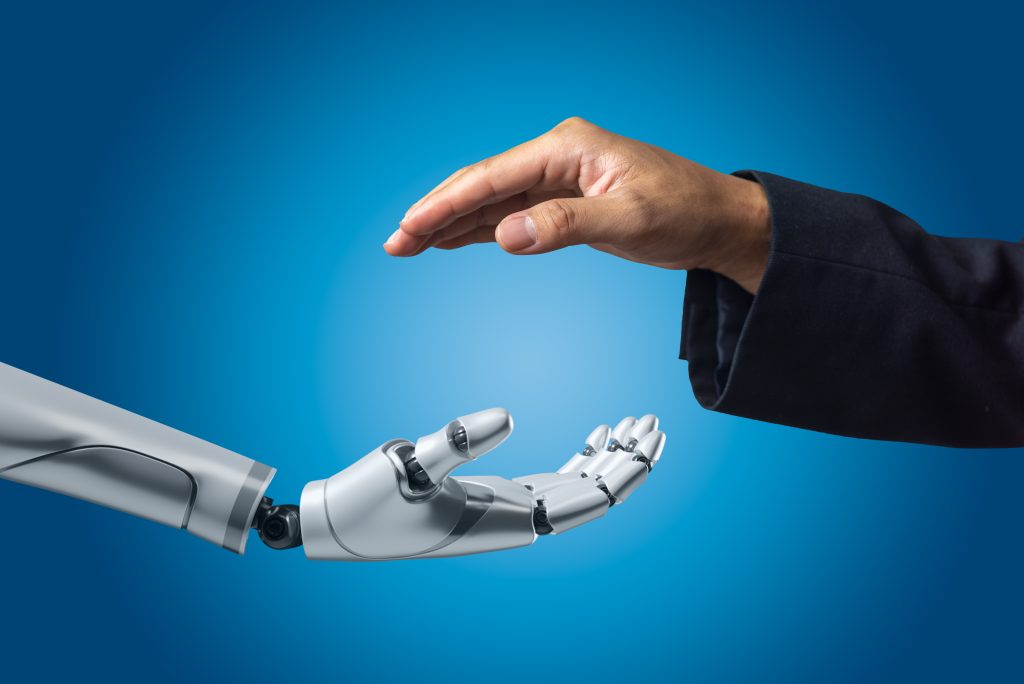
The implementation of long-term AI systems powered by Kalman filters holds transformative potential across several domains:
- Education:
- AI tutors capable of tracking student progress over semesters, tailoring lessons to individual needs while fostering intercultural competence.
- Facilitating long-term faculty development programs by providing insights into teaching practices.
- Global Business:
- Equipping AI systems with the ability to learn and adapt across cultural contexts, enhancing global customer service and team collaboration.
- Providing culturally sensitive recommendations for leadership and organizational development.
- Ethical AI Development:
- Promoting fairness and accountability by enabling AI systems to evolve ethically in response to intercultural challenges.
- Creating a robust feedback loop where human-AI interactions inform system improvements.
Challenges and Considerations
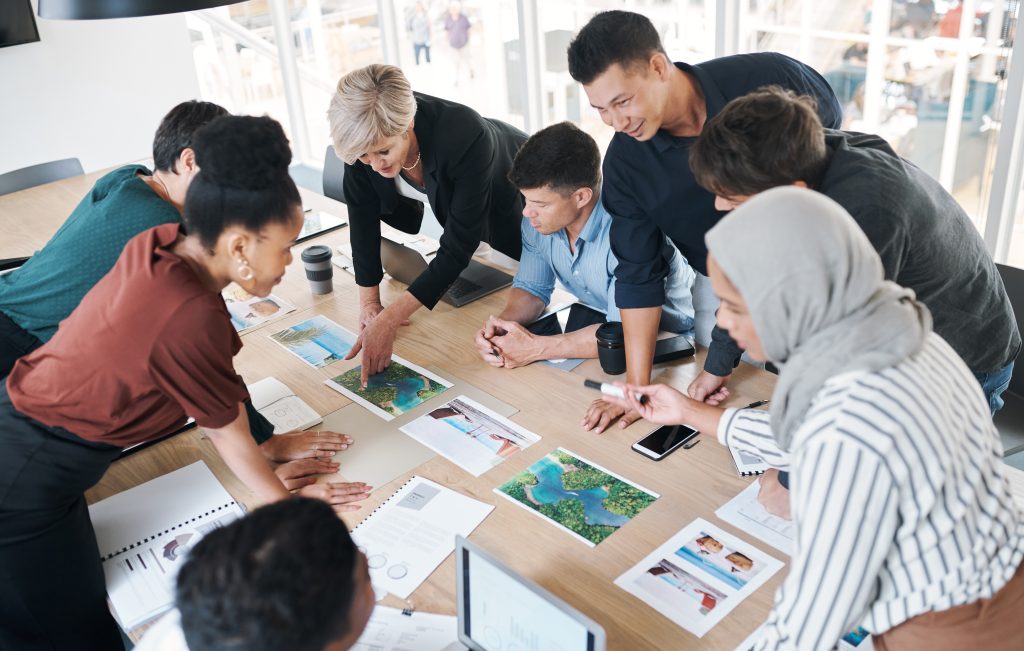
While the potential is immense, several challenges must be addressed:
- Data Privacy: Long-term storage of user data requires strict compliance with privacy regulations.
- Bias Mitigation: Continuous learning systems must be designed to avoid reinforcing biases.
- Infrastructure Needs: The implementation of Kalman filters at scale requires significant computational resources and advanced modeling techniques.
Conclusion: Toward a Smarter, More Culturally Adaptive AI
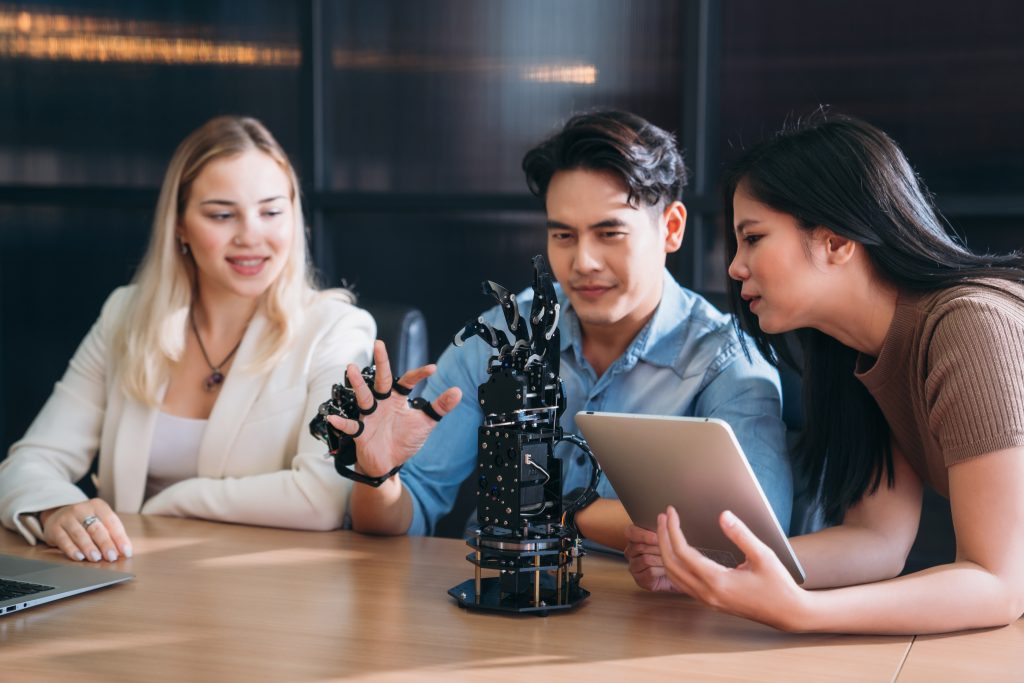
By integrating ICCSv2 metrics with modernized Kalman filters, we envision a future where AI systems are no longer constrained by short-term limitations. Instead, they become partners in fostering intercultural competence, ethical behavior, and personalized learning.
The transition to long-term AI systems represents not just a technological innovation but a paradigm shift in how we design, interact with, and benefit from AI. As educators, developers, and policymakers, we have an opportunity to redefine AI’s role in higher education, global citizenship, and beyond.
The time to act is now. The question is no longer whether AI can evolve but how we can guide its evolution to meet the complex needs of our interconnected world.
Call to Action: Share Your Thoughts!
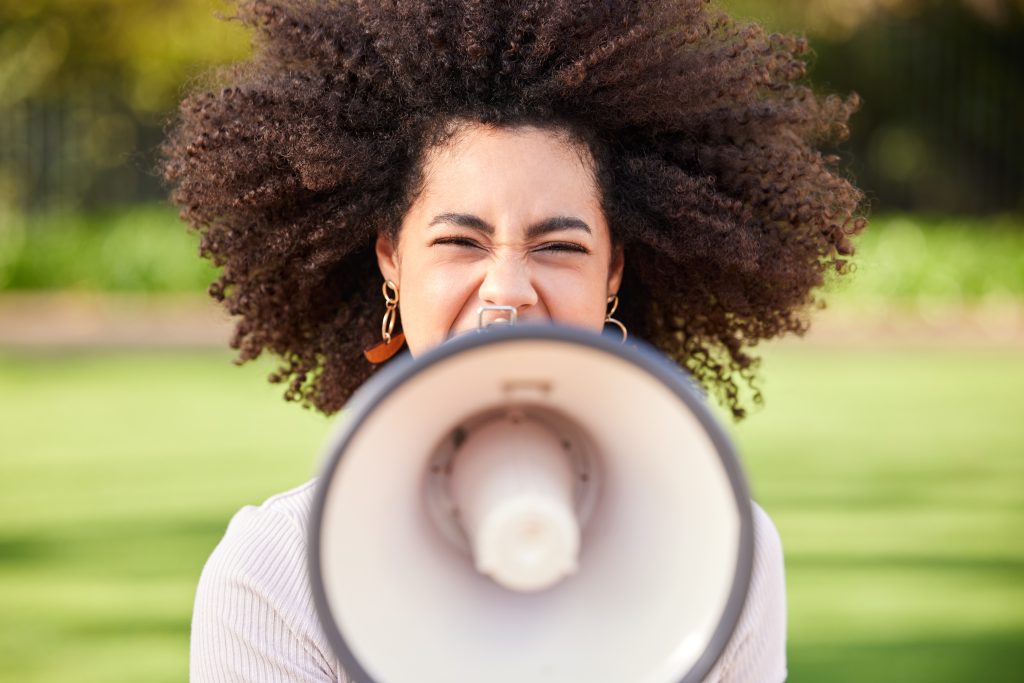
What are your perspectives on the potential of long-term AI systems? How do you see modernized Kalman filters shaping AI’s future? Leave a comment below or share this article with your network to join the conversation.